16S/18S/ITS Amplicon Sequencing and Transcriptomics
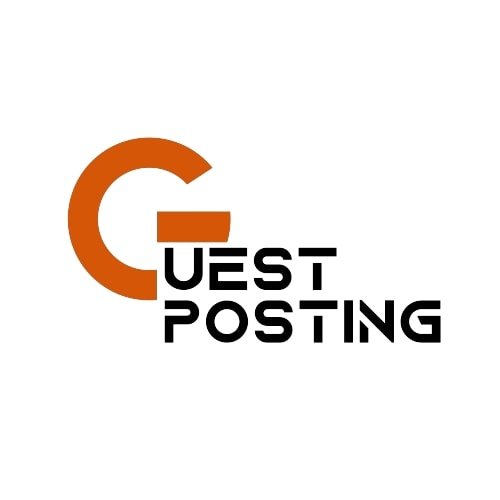
16S/18S/ITS Amplicon Sequencing
Microbial diversity sequencing, also known as amplicon sequencing, leverages next-generation high-throughput technologies to sequence gene sequences like 16S rRNA/ITS. This method allows for the simultaneous detection of dominant, rare, and unidentified species in a sample. It provides insights into the composition and relative abundance of microbial communities within the sample.
Transcriptomics
The transcriptome, encompassing all RNAs transcribed by a specific species, tissue, or cell type, is studied through high-throughput sequencing. This approach swiftly captures the entire set of transcripts in a particular cell or tissue, aiding in gene structure and function analysis, variable splicing, and the prediction of new transcripts. Additionally, it excels in detecting low abundance and novel transcripts.
Investigations into microbial-target organ relationships, such as the brain-gut and liver-gut axes, are prominent. Integrating microbial and target organ transcriptomes allows for a comprehensive understanding, linking microbial changes to transcriptional alterations and yielding valuable insights.
Microbial Diversity and Transcriptomics Analysis
Microbial diversity and transcriptomics multi-omics analysis strive to identify key biomarkers, suggest inter-sample relationships, and unveil biological significance by comprehensively considering both microbial and transcriptional data.
Our multiomics services and reports are divided into three main parts. The initial part evaluates multi-omics as a whole and assesses data quality. The second part focuses on identifying key marker genes, while the third part conducts correlation analyses to illustrate the level of correlation among distinct substances.
Cluster Analysis of Samples
The original cohort data underwent standard deviation normalization (Z-Score Normalization) and quantile normalization (Quantile Normalization) before being merged. Subsequently, dimensionality reduction clustering techniques were applied to visualize the interrelationships among samples, assess sample grouping, and gauge intra-group reproducibility. Two dimensionality reduction methods, namely PCA (unsupervised) and LDA (supervised), were selected. The outcomes following PCA dimensionality reduction were further employed in hierarchical clustering. Ultimately, linear fitting projection was utilized to illustrate variations among different omics within groups.
To further appraise the discriminative capacity of the multigrouping features in distinguishing sample groupings, a random forest model was constructed using the normalized multigrouping features. The model's classification performance was evaluated using ROC curves to determine whether the multigrouping features effectively predict distinct sample groupings. This random forest model also played a pivotal role in the subsequent section dedicated to biomarker screening.
Biomarker Screening
Employing a random forest approach, we assessed the significance of each substance within microbes and transcripts concerning the present subgroup. Higher importance scores suggest that a substance is more likely to serve as a biomarker distinguishing the current subgroup. The top 30 biomarkers, ranked by importance, were chosen to reconstruct the Random Forest model. ROC curves were generated through cross-validation involving 20 random permutations.
Each permutation involved dividing the data into a training set and a validation set (1:1 ratio). A random forest model was constructed using the training set and then applied to predict the validation set. In cases with more than two sample groups (>2), the micro-averaging method was employed to convert multi-classification results into binary classification. The effectiveness of the model's classification was assessed by the area under the ROC curve, where a larger area signifies a superior classification effect.
Correlation Analysis
Examining material differences among various omics through correlation analysis unveils inter-histological associations. Initially, we independently scrutinized microbial and genetic data, focusing on the top 1000 data entries with the most substantial absolute value of log2 (FoldChange). This selection was made while ensuring adherence to the significance criteria from the original single-omics analysis of differences. In instances where the dataset was less than 1000, all entries were included.
It's noteworthy that, when comparing across multiple groups, the screening process adhered to the significance (p-value) derived from the original difference analysis. Subsequently, pairwise correlation coefficients were computed for all data entries of microorganisms and genes.
What's Your Reaction?
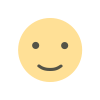

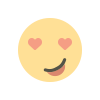



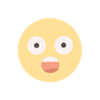