An examination of the ethical considerations involved in data analytics

Introduction to Data Analytics and Ethics
Data analytics has become a pivotal component in driving decision-making across various industries, including finance, healthcare, marketing, and government. By leveraging vast amounts of data, organizations can uncover insights, predict trends, and improve operational efficiencies. However, with great power comes great responsibility, leading to the essential need for ethical considerations in data analytics. Ethics in this context refers to the moral principles that govern how data is collected, analyzed, and utilized, ensuring that the rights and dignity of individuals are upheld.
As data analytics continues to evolve, so does the landscape of ethical dilemmas associated with it. From privacy concerns to algorithmic biases, these challenges can significantly impact individuals and society at large. Ethical data analytics not only fosters trust among stakeholders but also promotes accountability and transparency in decision-making processes. By addressing these ethical concerns, organizations can mitigate risks and enhance their reputation while ensuring compliance with regulatory standards.
Data Privacy and Confidentiality
Data privacy and confidentiality are among the foremost ethical considerations in data analytics. With the increasing volume of personal data being collected, the potential for misuse or unauthorized access has also risen. Compliance with data protection regulations, such as the General Data Protection Regulation (GDPR) and the California Consumer Privacy Act (CCPA), is crucial for organizations. These laws impose strict guidelines on how personal data should be collected, processed, and stored, emphasizing the need for consent and transparency.
Maintaining confidentiality involves safeguarding sensitive information to prevent data breaches, which can lead to severe consequences for both individuals and organizations. Ethical data analysts must implement robust security measures, such as encryption and access controls, to protect data. Furthermore, organizations should foster a culture of ethical data handling by training employees on privacy best practices and emphasizing the importance of responsible data use. By prioritizing data privacy and confidentiality, organizations can build trust with their customers and stakeholders while navigating the complexities of data analytics.
Informed Consent
Informed consent is a cornerstone of ethical data analytics, emphasizing the importance of transparency and respect for individuals' autonomy when collecting their data. It involves providing clear, understandable information about how data will be used, allowing individuals to make informed decisions about whether to participate in data collection processes. However, obtaining informed consent presents challenges, particularly in complex data analytics projects where the scope of data use may not be immediately apparent to participants.
One significant issue is the difficulty in articulating the potential uses of data in a rapidly evolving technological landscape. Organizations must strike a balance between being comprehensive and accessible in their explanations. This can be particularly challenging when dealing with big data, where data points might be aggregated and analyzed in ways that are not explicitly stated at the time of consent.
Best practices for obtaining informed consent include using plain language, offering examples of data usage, and ensuring that individuals can easily withdraw consent at any time. Additionally, organizations should regularly review and update consent processes to reflect changes in data practices or legislation.
Bias and Fairness in Data Analytics
Bias in data analytics can manifest in various ways, from the data collection process to algorithmic outcomes. Biased datasets can lead to unfair and discriminatory practices, affecting marginalized groups disproportionately. For instance, if a hiring algorithm is trained on historical data that reflects biased hiring practices, it may inadvertently perpetuate those biases, resulting in unfair hiring outcomes.
Addressing bias and promoting fairness in data analytics is essential for ethical practice. Organizations should implement strategies to identify and mitigate bias throughout the data lifecycle. This includes conducting bias audits, ensuring diverse representation in datasets, and utilizing fairness-aware algorithms that strive to minimize bias in decision-making.
Moreover, fostering a culture of inclusivity within data analytics teams can contribute to more equitable outcomes. By involving diverse perspectives in the data analysis process, organizations can better recognize and address potential biases, ultimately leading to fairer and more ethical data-driven decisions. This proactive approach not only helps in complying with ethical standards but also enhances the overall credibility and reliability of data analytics initiatives.
Transparency and Accountability
Transparency and accountability are vital ethical considerations in data analytics, ensuring that organizations operate in a responsible and trustworthy manner. Transparency involves openly communicating methodologies, data sources, and analytical processes to stakeholders, which helps build public trust. By providing insights into how data is collected, analyzed, and interpreted, organizations can foster a culture of openness and collaboration.
Additionally, transparency is crucial in algorithmic decision-making. When algorithms are used to make critical decisions, such as loan approvals or hiring choices, it is essential for individuals to understand how these algorithms function and the data that informs them. Organizations should strive to provide clear explanations of their algorithms and the factors influencing outcomes, enabling stakeholders to hold them accountable for their decisions.
Accountability goes hand-in-hand with transparency. Data analysts and organizations must be responsible for their actions and decisions, ensuring that they adhere to ethical guidelines and legal regulations. Establishing clear lines of accountability helps organizations manage risks associated with data misuse or unethical practices. This can be achieved by creating internal oversight committees or appointing data ethics officers to oversee data analytics initiatives.
Ethical Use of Data for Decision-Making
The ethical use of data in decision-making is paramount for organizations leveraging analytics to influence significant outcomes. When data-driven insights inform decisions that affect individuals or communities, ethical considerations must be at the forefront. Organizations should evaluate the potential impact of their decisions, weighing the benefits against possible harms.
For instance, while targeted marketing can increase sales, it may also lead to the exploitation of vulnerable populations. Therefore, organizations must adopt a balanced approach, ensuring that their use of data aligns with ethical standards and social responsibilities.
To promote ethical decision-making, organizations can implement frameworks that guide data use. These frameworks should prioritize fairness, accountability, and the protection of individuals’ rights. By establishing clear ethical guidelines, organizations can navigate the complexities of data analytics, ensuring that their practices are responsible and equitable while fostering trust among stakeholders.
The Role of Data Governance
Data governance plays a crucial role in ensuring ethical data analytics practices within organizations. It refers to the framework of policies, procedures, and standards that govern data management and usage. Effective data governance helps organizations maintain compliance with legal regulations, protect sensitive information, and uphold ethical standards.
Key components of data governance include data quality management, data stewardship, and clear accountability structures. By establishing these frameworks, organizations can mitigate risks associated with data misuse and enhance trust among stakeholders. Furthermore, a robust data governance strategy ensures that data analytics initiatives align with the organization's ethical values, fostering a culture of responsibility and integrity. Ultimately, prioritizing data governance is essential for promoting ethical data practices and achieving sustainable success in data analytics.
Conclusion
As data analytics continues to transform industries, the ethical considerations surrounding its use are more important than ever. Ensuring data privacy, obtaining informed consent, addressing bias, and promoting transparency are essential steps that organizations must take to build trust and maintain accountability in their data practices. By prioritizing ethical standards in data analytics, companies not only comply with legal requirements but also foster a culture of responsibility that benefits both individuals and society. For those looking to deepen their understanding of ethical practices in data analytics, enrolling in a data analytics course in Noida, Jaipur, delhi, goa, etc, can be an excellent opportunity. Such courses typically cover the essential concepts of data ethics, privacy regulations, and best practices, equipping participants with the knowledge and skills needed to navigate the ethical landscape of data analytics effectively. By gaining expertise in this field, professionals can contribute to creating ethical frameworks that guide responsible data use, ensuring that data analytics serves as a force for positive change in society.
What's Your Reaction?
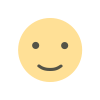

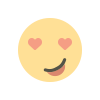



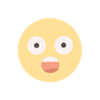