Fundamentals and Applications of Generative AI

The niche known as Generative AI has the impact that it has because of the progress present in the field of artificial intelligence. Generative AI, which can be viewed as one of the subfields of AI, aims to create new content and information, which is more often than not very close to what humans can create.
The use of Generative AI in business processes signifies a major step towards automation of processes coupled with improved decision making. Through the automation of mundane tasks and generation of new ideas, AI with applications of generative AI used in specific fields is helping companies to improve efficiency and to create new business strategies.
Understanding Fundamental Concepts in Generative AI
Generative AI sits at the boundary of creativity and technology as it is driven by rich datasets that can spur the creation of new content with the use of algorithms. This section examines language generation that has been motivated by the emergence of technical advancement of Generative AI. These concepts help us to understand the foundation models and tell us about “What is Generative AI?” exactly.
Understanding Generative Models
Generative Models are the main focus of Gen AI. Such models can be differentiated from regular predictive models in its ability to not just examine data but rather synthesize new data instances. This ability resembles the so-called creativity in humans since this type of system learns from reality and seeks to come up with something new that is similar in nature.
Deep Learning and Neural Networks
These features within generative models include deep learning and neural networks. Neural networks, usually deep ones, contain a body of connected nodes that are organized in layers in a format that seeks to bring out the functions of the human brain. One such ability is deep learning wherein these types of networks learn complex structures from a lot of supplied data.
Training and Fine-Tuning of Models
The planning and fine-tuning of models is a crucial part when it comes to the efficiency of the Generative AI model. This procedure is well detailed in exposing the models to wide datasets. More additional fine-tuning contributes heavily to the accuracy and effectiveness of a model making it responses to tasks or industries in which it is practiced.
Role of data
The role of data is very critical since it is what drives Generative AI and more so becomes the primary energy source. The outputs these models produce to their end users contain quality, effectiveness and legitimacy, and therefore depend upon the inputs data amount, type and its variability. This indicates why it is important to have large and diverse datasets for training.
Exploring the Inner Workings: Applications of Generative AI
Studying the technological intricacies of generative AI applications reveals the complex mechanisms that enable these advanced systems to function. This section provides a thorough analysis of the technical components of Gen AI applications, with a specific emphasis on the essential technologies, algorithms, and operational frameworks that serve as their basis.
Architectures of Neural Networks
Neural network architectures, such as Convolutional Neural Networks (CNNs) for image-related tasks and Recurrent Neural Networks (RNNs) or Transformers for sequential data like text, play a crucial role in Gen AI applications. These networks partially replicate the functioning of the human brain and play a vital role in the AI's capacity to acquire knowledge and make choices.
Training and Learning Algorithms
Gen AI apps employ advanced algorithms that gather information from large datasets through training and learning procedures. The learning process involves training the AI using extensive datasets, which can be categorized as supervised, unsupervised, or reinforcement learning. This training allows the model to make predictions or create new material by utilizing learned patterns.
Data Processing and Management
Efficient data handling necessitates the use of data processing and management. This involves performing preprocessing on data, handling missing or disorganized data, and ensuring the integrity of the data. The effectiveness of the AI is directly linked to the quality and quantity of the data it is trained on.
Optimization and Regularization Techniques for Models
Model optimization and regularization are employed in Gen AI systems to enhance efficiency and address overfitting. These systems utilize various techniques such as gradient descent and regularization approaches. These strategies help optimize the model to enhance its generalization ability and improve performance.
Transfer Learning and Model Adaptation
This may be useful in tasks where the acquired knowledge is utilized in performing another task well known as transfer learning. This realization means that a model that has been trained up on a certain task may have its training parameters adapted for the new task. More specifically however, model adaptation implies the process of adjusting or changing an already existing model.
Scalability and Deployment
The use cases of these applications also provide the need of taking factors such as. For processes that include many actors on many scales, cloud computing and distributed systems are the answer in terms of where to gather the required computing horsepower and space. Also, though there is a need for these models to be applied into real-world contexts, this ought to be done properly to avoid inefficiencies and risks.
Interfacing and Integration
For the efficient functioning of the Gen AI applications, it is necessary that they be incorporated well within the other system structures. This also means building strong communications bridges, in the form of appropriate APIs and interfaces, between the AI system and other applications or services where efficient communication would be necessary.
Considerations regarding Ethics and Security
These, and other ethics and security related, essentials should be incorporated at the core of all hard and software dealing above technical issues. This covers those cases when the system which was given to the AI to take decisions on, has some inherent biases against certain groups or individuals.
What are the Practical Applications for Generative AI?
Such types of events originated first during the coronavirus pandemic and are attributed to the popularity of special video applications like zoom, Skype, etc. For the various features, several types of umbilical cords were devised:
- Using chatbots to enhance client support and provide help management.
- Using special computer software to hotspot and capture an individual interpreting physical likenesses.
- Enhancing the process of dubbing films and other audiovisual works into different languages.
- Tasks include replies to emails, the numerous dating profiles, CVs, and academic papers composition.
- Creating one of a kind realistic images in unusual styles.
- Creating videos that would effectively i͟n the marketing of products.
- Provide new drug molecules to be synthesized for testing.
- Building physical materials and constructions.
What are the Restrictions of Gen AI?
The limitations of generative AI have been evidently illustrated using the initial implemental practices. The ways employed for attaining the varied aims are the basic reason for many problems caused by generative AI. The topic of a brief summary versus a broad contextual explanation with selective sources to prove basic concepts would be easily grasped.
Nonetheless, the lucidity of the summary is bought at a price whereby the user is unable to ascertain where exactly the information was sourced from. As far as the development or use of a generative artificial intelligence application goes, the following limitations should be considered:
- Identifying the origin of the object is frequently vague.
- Examining bias in the primary sources may pose challenges.
- The presence of stuff that sounds realistic makes it more difficult to identify inconsistencies.
- Adapting to unfamiliar situations can be confusing.
- Results have the potential to minimize or underestimate bias, prejudice, and hatred.
Conclusion
It is evident that concerning Generative AI Domain, this area is a blend of the latest technology and the greatest creativity. The investigation of the GAI with fundamentals and applications of generative AI of the future from both practical and theoretical perspectives has allowed for an objective and thorough comprehension of the foundations, purposes, principles, and instruments that this space seeks to change.
What's Your Reaction?
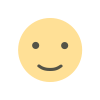

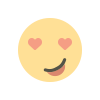



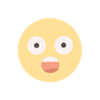